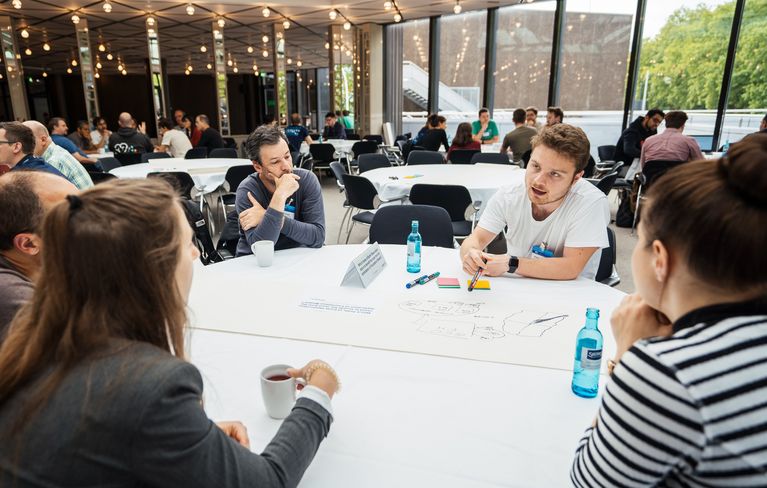
Helmholtz AI Conference 2024: a World Café Wrap
The AI World Café: Collaborative Conversations, held on June 14, 2024 at the Helmholtz AI Conference brought together a diverse group of participants for an engaging 90-minute session providing a unique platform for open dialogue, idea sharing, and collaboration.
Participants formed small groups of up to 10 and rotated through three 20-minute rounds of vibrant discussions, covering both predefined and spontaneous AI-related topics. This dynamic format encouraged deep dives into various subjects, allowing attendees to gain new perspectives, exchange insights, and foster meaningful connections within the AI community.
The session sparked thought-provoking conversations and offered valuable opportunities for professional networking and collaborative thinking. We would like to share our insights with you - enjoy our World Café report below!
-
The Gender and AI World Cafe session focused on the intersection of gender issues and artificial intelligence. The discussion aimed to address the societal dangers of recent AI advances, particularly for women and minorities. Bias in AI models was discussed, as well as diversity and inclusion in the AI workplace, and how the two are related.
All participants agreed that a diverse and inclusive workforce is key to attracting the best talent, gathering a variety of perspectives, fostering creativity, and preventing blind spots in the development of AI solutions, as well as in science in general. We should not tire of pointing out the benefits of diverse and inclusive work cultures, not only because they are more fair and equitable, but also because they lead to better team performance and better research.
Many different ideas and aspects were discussed on how to achieve a better gender balance in the field, e.g. through role models, outreach activities in schools and awareness raising. Open questions were how to reward and value activities in this area within academia and how the German situation differs from other countries. The Helmholtz AI conference itself was identified as a positive example, with a high number of women attendees and speakers and inclusivity measures such as childcare.
One of the major problems with AI systems in production has been identified as unchecked bias in models related to sex, gender, or other attributes. Bias cannot be completely avoided and can be introduced at every step of the model lifecycle, from data collection to deployment. The Helmholtz AI conference highlighted some interesting contributions in the area of bias detection and mitigation. In addition to ongoing research in this area, it is important to educate the general public as well as researchers to be aware of the limitations of AI and to be sensitive to potential problems. Generative AI poses a unique risk due to its lack of transparency and our tendency to humanize its output.
Clear rules and incentives for responsible AI development are needed and must be enforced. The European AI Act is a step in the right direction.
As a concrete outcome, we identified the need to train researchers in the general area of AI ethics, for example in the form of a course at the Helmholtz Information & Data Science Academy (HIDA), possibly in cooperation with Helmholtz AI ethics advisors. The World Cafe established a first connection between relevant stakeholders.
-
Introduction
During our table discussion, we delved into the topic of Hybrid models and other related topics such as simulation-based inference SBI. The discussion was initiated with an introductory statement stating what it means and highlighting the importance and relevance of the subject in today's AI model context. A Hybrid model can simply be defined as the combination of conventional model, also known as data driven model with some form of differentiable physical model(s), which can also be called a model-based method. The idea is simply to have prior knowledge infused in this model-based method and allow ML method to handle the part that we do not understand. This idea is borne out of the fact that certain dynamic problem cannot be fully resolved by only data driven approaches, for example in a noisy setting, while some certain model-based methods can be over-simplistic. The other advantage of this is the ability to capture and understand the causality behind the problem. This idea is also believed to aid research and ingenuity.
Different ideas on Hybrid Model
- The Neural Network architecture in hybrid model is not pretrained and, it is conditioned on the physics equation to learn one or more parameter in the physics simulation or an Ordinary Differential Equation (ODE) based on the input data.
- The Hybrid model can also be set up in a stochastic sense, which is very good for dynamic settings / task. Therefore, uncertainties can also be extracted from the model.
- A good example of adding stochasticity to a Hybrid model could be addition of Stochastic Differential Equation (SDEs) in replacement of ODEs to achieve the stochasticity of the model, which in turn allows us to model uncertainty and noise.
- There can be stochasticity in the parameter but also in the model process. Hence, the SDEs can help to model stochasticity in the dynamic process.
Identified bottleneck
The idea behind Hybrid model sounds nice, however there are still a lot of bottlenecks involved, these include but not limited to:
- Expensive model-based system/simulation: certain models do take time to run and sometimes require a lot of computational resources
- Cross platform compatibility: There are situations where these algorithms are located on another platform or written with languages e.g., FORTRAN while the ML model is located on another platform, say Python. This is a problem because computing gradients will be difficult.
- The effect of insufficient or poor/noisy data into the model-based method.
- How to accurately implement the Hybrid model: this is important because there are many ways to implement Hybrid models.
- How sure are we that the model-based method / algorithm encapsulates the problem we want to solve?
Controversial Points
The use of optimal transport to speed up computational time and maintain the ability to obtain good accuracy: this idea was pitched and discussed, but fellow members indicated that it is counter-productive because it essentially will result into the same issue
Key Ideas to solving the bottlenecks
Several key ideas emerged from our discussion to solving some of these issues, if not all. They include:
- Moving the simulation and Neural Network into JAX for example where features like Just In Time (JIT) compilation method can be implemented to significantly accelerate the execution of these ODEs and have them differentiated.
- Looking into MOSCOT (Multi-omic single-cell optimal transport tools) for better ways to implement optimal transport tools.
- Looking into POT (Python Optimal Transport) for better ways to implement optimal transport tools. However, this is faster and can be easily used with PyTorch existing framework.
- Proposing other hybrid model architecture such as Aphynity as an example of Hybrid model flavour.
- We should start all hybrid model first from a toy dataset perspective and see how the Neural Network architecture recovers the underlying pattern / information of the pre-defined problem.
Potential Follow-Up
To build on our discussion beyond the conference, the following was initiated:
- Sharing of contacts among those that are interested in future collaborations.
Summary
The intensity of our discussion suggests that there is a strong interest in Hybrid model and other related topics such as simulation-based inference. We had a very wide range of participant which include very knowledgeable members who have done research on this topic and participants who are just getting ready to delve into the space of Hybrid model. Over all, it was fun and impactful.
-
Effectively transitioning AI research innovations into practical, operational AI models that deliver tangible value is a critical challenge. Often, a research paper marks the end of a project, but there's potential for much more. Creating a website, developing a Python library, or securing a patent are just a few ways to extend the impact of research, benefiting the broader community.
Consensus and Controversy
Consensus: People agreed that it can be helpful to them and their clients and adds credibility to your work because it happens that you are not able to produce the results of a research paper as they had promised in the paper. But with this people can see your working so it adds credibility to the work you have done and it also gain you a point in showcasing your work easily to gather more work in future.
Controversy: The most controversial aspect was the misconception that transitioning research into practical applications would replace core research activities. Participants worried about the additional time commitment. However, we clarified that this effort is meant to complement existing research, not replace it. We agreed that researchers can focus on their primary work while seeking help from specialized teams to develop application-ready products. This way, they can publish their work and also contribute to practical applications.
Key Ideas
We brainstormed various ways to make research projects tangible. Tangibles could include a website, a software project proposal, a software library, or a patent. We showcased our projects, such as Operational CYGNSS, freva-gpt, drift detection, and video whale detection, to illustrate these concepts.
We discussed how to determine if a project is suitable for transition. This involves researching the demographics of the project's potential users. If coders are the primary users, then creating a software package, library, securing a patent, or writing a software proposal is appropriate. If the project is for non-coders, then a user-friendly website is more suitable. Researchers can approach our team (DKRZ AIM consulting) or KIT team (SCC) for help.
Collaborating with the industry was also one point. We need to ensure to talk to multiple companies through your network or at conferences. Find someone who is ready to collaborate with your idea, better show that a small proof of concept. This way you can get them interested in your idea and making sure that projects converted into something bigger. Go to HIFIS, they also have a course which you can join for getting started with your own company.
At the very least, if you think your project does not qualify for any of the above; once you are done you want to ensure that your product can be easily used by others. To do that, you can use the Imagine-AI marketplace (https://dashboard.cloud.imagine-ai.eu/marketplace) and contact the KIT team (SCC) so that anyone can just pull your projects and data and start using it without any trouble. This approach ensures your work is accessible and beneficial to others.
Follow-up
We encouraged participants to submit vouchers to us for these kinds of use cases. Many seemed enthusiastic about the prospect of receiving help in this direction. We look forward to supporting researchers in making their work accessible to everyone.
-
We discussed how AI researchers in Helmholtz can cooperate. Originally, we intended to identify options for Helmholtz AI research units to cooperate but quickly decided to widen the scope to all researchers in Helmholtz working on AI.
We identified that people need platforms to network, common problems to work on, and incentives to work together.
Common problems can likely be best worked on when common data sets exists together with a platform to share the data. However, there does not seem to be a strong incentive for openly shared data sets with well-maintained metadata.On the networking side, Helmholtz AI already offers the Associate status, the Food for Thoughts seminar series and the Mattermost. Unfortunately, few of the discussing participants knew of these options. Hence, the existing options might need more advertisement. Helmholtz AI, its research groups and consultants might need to become more visible to applied AI researchers at the different centers.
To facilitate networking, we came up with the idea to have a “project” or “paper” matchmaking as part of HAICON25: Connect people from different institutes within and beyond Helmholtz to work towards joint publications, followed by potential (Helmholtz AI) project applications.
-
Generative models have a wide range of potential applications for supporting medical image analysis, including increasing the resolution of medical images, filling in missing data, generating synthetic data, or generating other imaging modalities.
While the potential of these methods is undisputed, we here asked ourselves and discussed what risks would be associated with the applications of generative models for medical image analysis, and how they can be addressed in practice.
As our main result, we came to the conclusion that the evaluation, continuous monitoring, and communication of generative models and generated data is crucial to justify their application in medical applications.
We think that the question on whether a generative model should be used or not can only be answered given a specific application context that allows quantifying the task-specific performance of a model.
For example, we see the application of generative models as mostly uncritical when it comes to visualization or education, as long as generated data is clearly communicated and marked as such.
To the contrary, we would currently advise against the use of generative models in the context of safety and health critical applications.
-
The discussion at our table focused on the potential and challenges of collaborating with industry partners in the field of AI. The conversation started with an acknowledgment of the opportunities these collaborations present, particularly in addressing specific problems and gaining access to valuable data.
Key Points of Discussion:
Opportunities: Participants agreed that industry collaborations offer significant benefits, such as the possibility of joint research projects, AI consulting, and funding opportunities that are often harder to secure through purely academic research. These partnerships also provide practical avenues for PhD students to engage in industry-relevant work.
Challenges: A major point of concern was the fear that data promised by industry partners might not be shared as agreed upon, and that academic researchers may find themselves obligated to complete tasks they no longer wish to pursue due to contractual commitments. There was also concern about potential non-scientific practices from industry partners, which could lead to misleading claims.
Marketing Needs: When offering AI consulting services, participants emphasized the importance of effective marketing to increase visibility and attract potential industry partners.
Follow-up Actions
The discussion highlighted the need for clearer communication and well-defined agreements between academic and industry partners to mitigate risks. There is also an interest in developing strategies to enhance the marketing of AI consulting services to better connect with industry needs.
-
A digital twin is a computer model that represents a real-world physical object, system, or process. It acts as a nearly indistinguishable counterpart of the physical entity, allowing for simulation, testing, and maintenance. Recently, there has been a growing trend of funding calls for projects focusing on digital twins of various aspects of the natural world. Notably, the European Space Agency's (ESA) initiative, Destination Earth, aims to build a "highly accurate digital twin of the Earth." Such a model would likely incorporate satellite observations and cutting-edge machine learning techniques, including foundation models specifically designed and trained for this purpose.
While the role of digital twins in engineering is fairly clear, their scientific utility is more nuanced. To explore this, we held a discussion to define what "digital twin" means within the contexts of Earth sciences, physics, medicine, Earth observation, and machine learning, as well as the unique challenges it presents in each of these fields.
One key consensus was that what distinguishes a digital twin of something like the Earth from that of an airplane is the level of control one can exert over the system. Without a clear feedback loop, the distinction between a digital twin and traditional simulation models driven by observation becomes less apparent. In engineering, a major benefit of digital twins is the ability to test various scenarios. However, in the case of Earth, validating such scenarios is challenging due to the limited historical data available, and the inability to artificially induce many scenarios for testing purposes.
Another challenge is the vast amount of data a digital twin of Earth would generate, making it prohibitively expensive to process on the scale required. Thus, the software and computational demands for a digital twin of such a large and complex system can be overwhelming.
Looking for the original notes?
Find the analog notepads from the World Café below!
Analog notepads from the World Café:Subscribe to our newsletter
Stay up to date with all things AI in science across the Helmholtz Association.
Subscribe