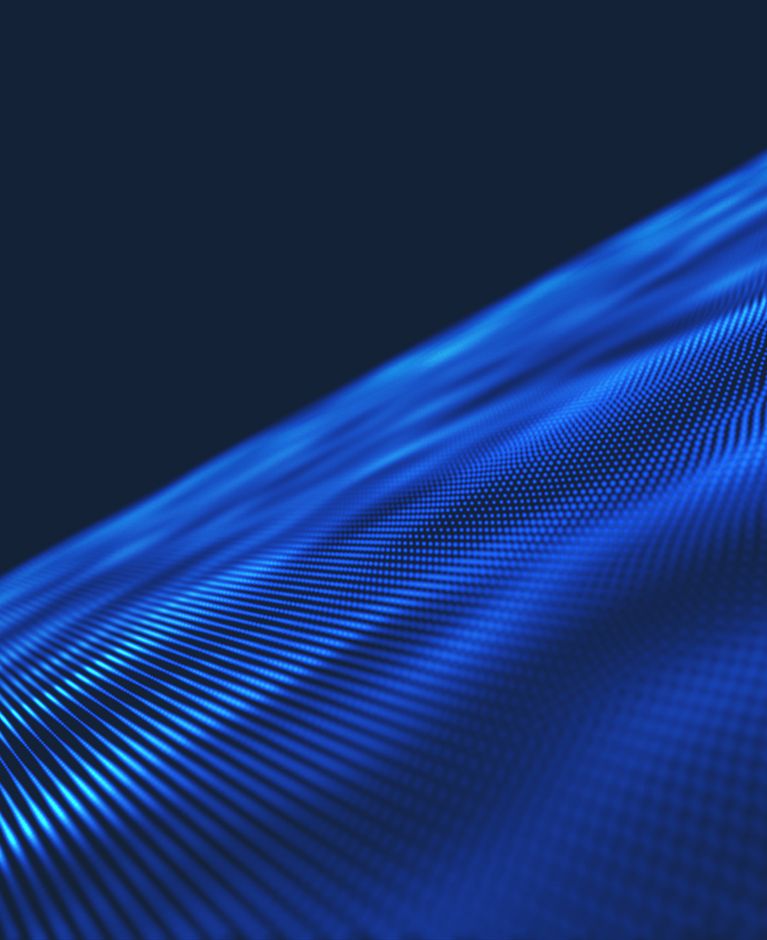
Molina Team // Aeronautics, Space and Transportation
Daniela Espinoza Molina - Team leader
HELMHOLTZ AI CONSULTANTS @ GERMAN AEROSPACE CENTER
AERONAUTICS, SPACE AND TRANSPORTATION-FOCUSED AI CONSULTANTS
The Helmholtz AI consultant team @ DLR provides expertise from Earth observation, robotics and computer vision and an HPC/HPDA support unit.
The team covers a comprehensive package of AI-related services. It is supposed to support both projects within LRV as well as cooperative projects with other Helmholtz centres.
The team
SELECTED ONGOING VOUCHER PROJECTS
Our most prominent current challenges.
-
- Challenge: In order to achieve a more virtual design and certification process of jet engines in aviation industry, the uncertainty bounds for computational fluid dynamics have to be known. In our in-house CFD solver TRACE, we have already implemented the physically motivated, data-free forwards approach of perturbing the eigenspace of the Reynolds stress tensor. This method tries to estimate the uncertainty bounds by conducting five distinct simulations, belonging to five extremal states of the Reynolds stress tensor. First testing yields the overprediction of the uncertainties of the turbulence model in certain flow regions, where the baseline model is able to accurately predict the quantities of interest. The root cause for this might be, that the perturbations, in terms of the extremal states of the Reynolds stress tensor, do not vary in space of the computational domain.
-
- Challenge: With increasing global freshwater scarcity, universal access to clean water and sanitation has become a key societal challenge. In many developing countries, rapid urbanization and the continued expansion of slum areas exacerbates this challenge. Unregulated development and decentralized coping behaviors in slums overwhelms the planning capacity of authorities using traditional approaches. Earth Observation (EO) satellites provide a tremendous amount of highly accurate global environment geo-information, fostering the skyrocketing development of Artificial Intelligence (AI) based approaches in EO (AI4EO). The challenge is to model the commercial water demand and to do a water affordability analysis for slum households combining ground truth and EO data.
-
- Challenge: The main goal of this collaboration is to develop a forest type/density prediction system as a preliminary building block of our planned bio-mass estimation system.
-
- Challenge: The search for ever lighter vehicles is still a very topical objective nowadays. OEM’s legal restrictions concerning pollutant emissions, the need to reduce the average fuel consumption and to save raw materials are three key factors that drive engineers to explore innovative solutions to minimize vehicle mass. Mass reduction improvements, however, are not intended to endanger the safety of vehicle passengers. Despite the great accuracy in reproducing faithfully crashworthiness problems, FEA simulations cannot be integrated into structural optimization approaches based on conventional methods (such as Monte-Carlo) because of the high cost of crash simulations in terms of time and overhead. The challenge for the engineers is to integrate an effective and efficient structural optimization strategy in the Production-Development-Process (PDP) which can handle successfully these black-box functions (FEM solvers). Surrogate-Based Optimization strategy could be powerful method for these applications, provided that metamodels are tuned effectively and all the information are gathered properly. Especially when it comes to expensive black box functions such as crashworthiness applications, taking advantage of all available information is crucial. Within the iterative optimization process, every single point must be carefully chosen in order to gain new valuable information. At the same time, however, it is crucial to find a relationship between the various regression models, iteration after iteration. This is particularly relevant in order to obtain a robust model that can be eventually stored and fitted with new information whenever necessary. For this purpose, the choice of hyperparameters plays a key role and must be weighted across several iterations. Furthermore, since often all available data must be used for training the metamodel, common issues such as overfitting must be avoided. Therefore, the main goal is to investigate an appropriate Active Learning strategy suitable for crashworthiness applications, to unsure that relevant information is not lost along the way.
-
- Challenge: Rainfall-induced landslides are a major type of natural hazard that cause significant human and economic losses in mountainous regions worldwide. Proper assessment of hazard and risk posed by impending slope failures is fundamental to formulating proper strategies to mitigate environmental damage and related societal disruption. However, there are notable dynamic interactions due to climate change, active tectonics and increases in anthropogenic activities, such as deforestation, overgrazing, and urbanization. An automated detection system for early landslide identification and slope stability assessment is key in creating an effective landslide warning system. In this voucher, transfer learning should be explored to produce a convolution based semantic segmentation model to work with limited data.
-
-
Challenge: In this project, components of filamentous networks of the cytoskeleton (i.e. actin, tubulin and intermediate filaments) inside of mammalian cells shall be automatically detected and characterized in 2D fluorescence microscopy images. Changes between differently treated cells shall be automatically recognized and properties of the recognized structures automatically quantified. These filamentous structures are important for a vast variety of cellular functions, ranging from structural integrity, intracellular transport or sensing of environmental conditions, and signal transduction. Thus, filament networks play crucial roles in all mammalian cells. In microscopic images, filaments are visualized as many long, sometimes branched structures. Currently, these filaments are analyzed manually with an extremely high workload and limitations in the available sample count. An automated analysis would therefore be needed for most biological research areas that work with such image analyses and could further be adapted for other sub-cellular components.
-
SELECTED COMPLETED VOUCHER PROJECTS
Project highlights of our previous work.
-
- Challenge: The Institute of Vehicle Concepts (DLR) has several research domains, where a huge amount of data is generated. Currently, most researchers lack machine learning knowledge. Therefore, to start filling this gap, we would like to receive training, aiming to explain the requirements for input data, how data processing takes place, and which results could be expected. Also, we would like to have a series of workshops with the consultants to discuss potential applications, and prioritize them and start planning realization vouchers.
-
- Challenge: The Department of Gravitational Biology at the Institute of Aerospace Medicine (DLR) investigates human performance in extreme environments to gain deeper insights in physiology and develop new applications for manned space exploration and clinical practice. In this project, high resolution cardiovascular data will be used to find early markers and changes that could indicate a developing orthostatic instability or pre-syncope symptoms. Despite a long history of medical research, the underlying causes of syncope are unknown in half of the cases. The Institute has a large pool of cardiovascular data from tilt table or human centrifuge studies. Also, this data my be used in combination with data from bed rest induced deconditioning ageing effects. A practical application of such a solution can be found in wearable devices that continuously monitor cardiovascular data of risk patients as well as older people as preventive measure.
- Approach: To tackle this problem, we will design and train a model using this data to find early signs of performance drops and cardiovascular events before vasovagal symptoms occur.
-
- Challenge: One of the simplest and oldest ways to visualize fluid flow in experiments is the application of tufts. Tufts are small pieces of rope/wire that align themselves with the local flow direction. In regions of separated flow the tufts typically display a very unsteady behavior. Tufts or flow cones are used in both flight test and wind tunnel experiments to determine the local flow on a surface to improve the understanding of the flow and provide validation data for numerical models. The tufts/flow cones are filmed by a camera. Afterwards the images are processed in order to obtain the local flow direction in terms of angle, shape, temporal behavior, etc. of the tuft. In order to evaluate the temporal behavior of a tuft it is paramount to compare the status in one image to the status of the same tuft in another image. Thus, it is proposed to develop a fully automatic post processing chain for tufts, that should work for a wide variety of images from different campaigns (differences in lighting, perspective, contrast, background, etc.).
- Approach: The consultant team will design an implement a ML pipeline to detect and segment the tufts and cones in the raw images. Then, this information is going to be used to detect the direction, shape, and length of these elements.
-
- Challenge: One of the main topics of the Multiphase Flows and Alternative Fuels Department at the DLR is to investigate alternative aviation fuels to mitigate climate impact. Particularly, the Department develops physical-based models to predict the properties and performance of aviation fuels, which are a mixture of up to hundreds of different hydrocarbon species. As a new field, the prediction capability of different Machine Learning algorithms regarding fuels properties is investigated. The results so far are very promising. For some fuels and properties the applied AI algorithm shows even smaller errors than the quite sophisticated physical based models. Therefore, the Department is interested in studying the the prediction of model uncertainty.
-
- Challenge: The confluence of climate change, environmental protection and diminishing resources have promoted the development of low carbon footprint and sustainable technologies. The efficiency and functional capability of many engineering devices and industrial processes is strongly influenced by turbulent reacting multi-phase flows. Practical examples include (i) aeroengines where interactions of turbulent flow, fuel spray and combustion chemistry determine engine operability, thermodynamic efficiency and emissions, (ii) industrial sprays where an insufficient atomisation and droplet dispersion can impair product quality and yield and (iii) agricultural applications where an inhomogeneous distribution of herbicides and pesticides can lead to loss of crops or wastage and excessive water and ground degradation. Thus, the accurate description of interactions of turbulent flow, reaction chemistry and multiple phases is of paramount importance to develop clean and environmentally friendly technologies. This requires improved control and a more profound fundamental understanding. However, the advancement of the latter is impeded by the lack of quantitative data due to difficulties of automatically identifying physical and chemical states. State-of-the-art detection algorithms rely on predefined conditions that are cumbersome to develop and implement. A primary application of machine learning is the identification of features with common attributes in images. The objective of this voucher is to develop an automated image segregation tool. This tool should recognize physical and chemical states in scientific images. Thelong-term objective of related machine learning activities is the development of a general framework for the identification of physical and chemical states that accelerates quantitative data analysis. The efficient and reliable evaluation of data provides a competitive edge to research projects and offers new funding routes. Moreover, the data facilitate, in combination with the simultaneously measured turbulent flow field and additional scalars, a quantitative description of turbulence–chemistry–phase interactions and close the knowledge gap by advancing fundamental understanding. The acquired data will further support the development of more comprehensive numerical models, novel combustion concepts and industrial processes.
- Approach: The consultant team implement a segmentation network (Mask R-CNN) to learn to detect and segment targets in images. The instance segmentation results can be used for a quantitative understanding of turbulence-chemistry interactions.
-
- Challenge: The Propellants Department of the Institute of Space Propulsion (DLR) is investigating the combustion processes in a paraffin-based hybrid rocket engine under different operating conditions. More than 300 combustion videos have been recorded with a high-speed video camera. To process all this data the Department wants to develop an automatic analysis procedure to identify essential flow structures and different combustion phases. Particularly, the aim of this voucher is to implement and to evaluate advanced distance and dissimilarity measures (e.g., based on structural similarity).
- Approach: The team implemented a solution using HEAT, an Open Source Software package (https://github.com/helmholtz-analytics/heat) for distributed data analysis and machine learning developed by KIT and the DLR, based on k-means++ and spectral to identify short-term turbulences.
FEATURED PUBLICATIONS
-
-
Alexander Rüttgers, and Anna Petrarolo (2021). Local anomaly detection in hybrid rocket combustion tests. Experiments in Fluids 62, 136, doi: https://doi.org/10.1007/s00348-021-03236-1
-
Andrés Camero, Hao Wang, Enrique Alba, and Thomas Bäck (2021). Bayesian neural architecture search using a training-free performance metric. Applied Soft Computing 106, doi: https://doi.org/10.1016/j.asoc.2021.107356.
-
Yao Sun, Lichao Mou, Yuanyuan Wang, Sina Montazeri, and Xiao Xiang Zhu (2021). Large-scale Building Height Retrieval from Single SAR Imagery based on Bounding Box Regression Networks. ISPRS Journal of Photogrammetry and Remote Sensing, 18, pp. 79-95, doi: https://doi.org/10.1016/j.isprsjprs.2021.11.024.
-
Jongseok Lee, Jianxiang Feng, Matthias Humt, Marcus Gerhard Müller, and Rudolph Triebel (2021). Trust Your Robots! Predictive Uncertainty Estimation of Neural Networks with Sparse Gaussian Processes. In Conference on Robot Learning (CoRL). Proceedings of Machine Learning Research (PMLR), doi: https://doi.org/10.48550/arXiv.2109.09690
-
Lukas Kondmann, Aysim Toker, Marc Rußwurm, Andrés Camero, Devis Peressuti, Grega Milcinski, Pierre-Philippe Mathieu, Nicolas Longépé, Timothy Davis, Giovanni Marchisio, Laura Leal-Taixé, and Xiao Xiang Zhu (2021). DENETHOR: The DynamicEarthNET dataset for Harmonized, inter-Operable, analysis-Ready, daily crop monitoring from space. In Thirty-fifth Conference on Neural Information Processing Systems (NeurIPS) Datasets and Benchmarks Track (Round 2). PDF
-